When it comes to evaluating the effectiveness of a fertility treatment, like any treatment in medicine, experimental design is key. And never more than in studies where several variables influence the outcome, and the population is heterogeneous, as we often see in IVF. Ever heard of the saying ‘garbage in, garbage out’? If you start on the wrong foot, you will end on the wrong foot. In this case, the first foot has to do with study design, and in particular, sampling: what patients are you going to select for your study?
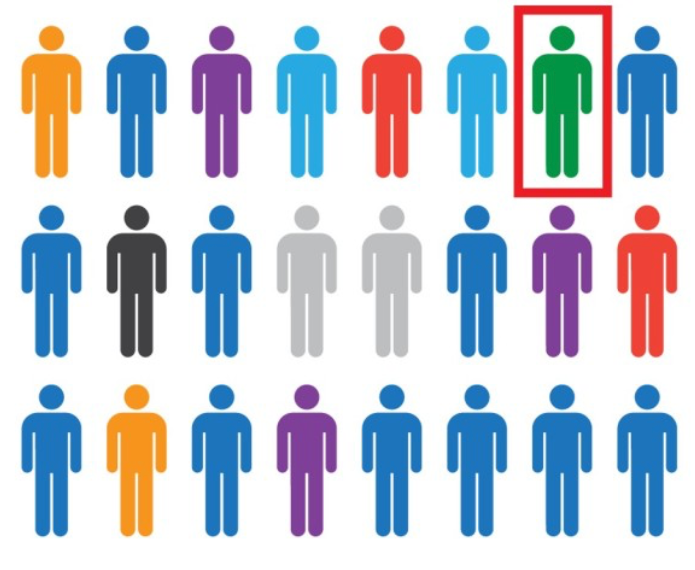
Dr. Art is frustrated with patients that have had multiple failed cycles, and has come up with a new endometrial treatment that he thinks might improve their implantation rate. He decides to test it. It is simple. He will recruit patients with multiple cycle failures, treat them and see if implantation rates improve! He makes some quick calculations regarding recent patients that might be interested in participating in the study. Should he use a group of women with 4 failed cycles? 3 failed cycles? A mix? If he treats a group with the worst prognosis and some of them get pregnant, what a result… He already sees his study gracing the cover of Human Reproduction…
In reality, if Dr Art sets up his experiment as planned, and finds some of the patients become pregnant after his treatment, the reviewers at Human Reproduction will send him flying. Why? because he set himself up for success: this patient group, in fact, will always give you better results, as it impossible to have worse results, and stochastically some women would get pregnant anyways. Statistically speaking, we are observing a phenomenon called regression to the mean, which implies that extreme values (like repeated failures) tend to move closer to the average over time. This means that some women who have had multiple unsuccessful IVF cycles may eventually succeed simply by chance. Using this group without comparison is likely to show improvement even if the new treatment had no real impact.
Dr Art’s experimental design is in urgent need of improvement. For starters, he needs a control group: a group of the same type of patients that will receive either a placebo or the standard regime of care. Second, he needs to consider his N. Third, he needs to think about confounding factors: age, BMI and any other factor he might consider relevant. Fourth, he must consider how he will assign patients to the treatment vs control group…
In sum, he needs to design his study and sample his population correctly!
Dr. Art may find his enthusiasm somewhat tempered after these considerations, as they imply he will need to enroll more patients, which are sometimes difficult to find in sufficient numbers, but getting on the cover of Human Reproduction is easier said than done... Before enrolling the patients, make sure to get your study design and sampling right!
Commentaires